
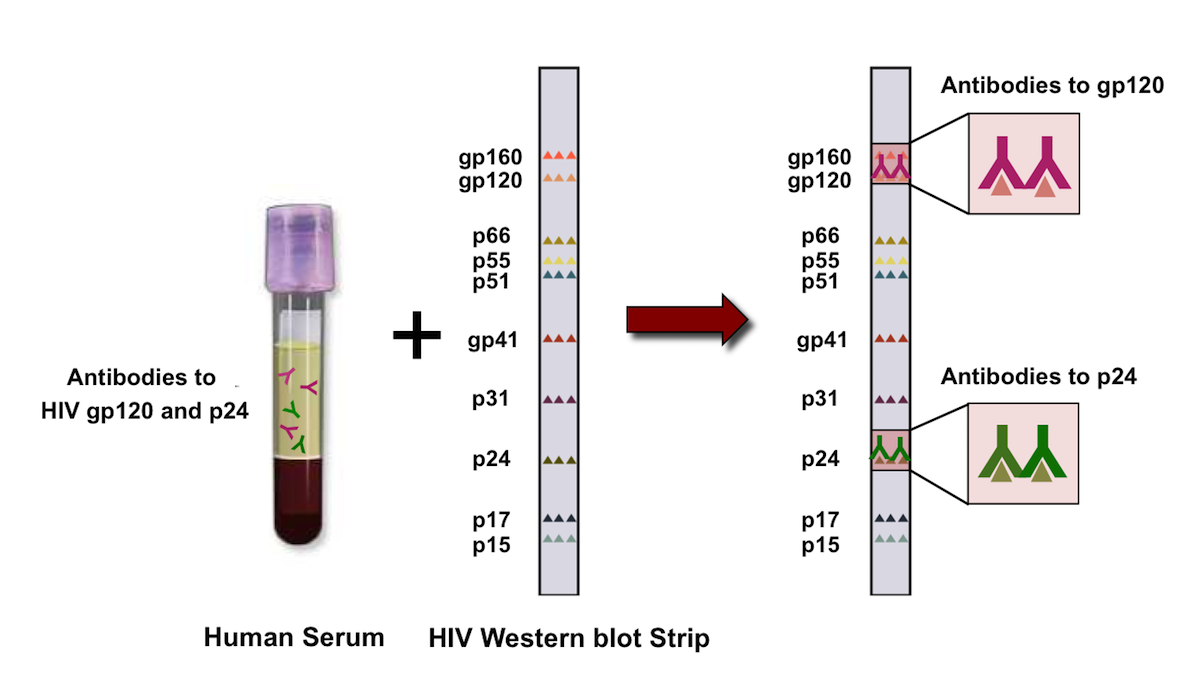
For example, assuming that the total amount of sample RNA is constant across arrays, the intensities are scaled such that the sum or the average of all intensities is equal across arrays (scaling methods ). In order to compare arrays quantitatively, several normalisation strategies have been proposed, where expression indices or intensities are scaled or transformed depending on the assumptions underlying each strategy. For microarrays, such as single channel oligonucleotide arrays, the issue of data normalisation arises naturally when expression indices, obtained from gene probe sets intensities, need to be compared across different arrays, for example to identify differentially expressed genes. Nonetheless we investigate linearity in our Results section, where we quantify the extent of the linear range in the case of two detection systems: enhanced chemiluminescence (ECL) with X-ray film and ECL with charge coupled device (CCD) imager.Īlthough the topic of data normalisation has been widely explored in the context of microarrays, it has not yet been fully investigated in the context of Western blotting. Because this method is highly labour consuming and is not a laboratory common practice, we do not consider this approach in this paper. These are dilution curves that need to be treated simultaneously to samples of interest, and in most situations constructing these dilution curves is not practical. To detect and correct non-linearity, the authors in suggest to use hyperbolic calibration curves to interpolate the correct relative concentration of the proteins of interest. Here we investigate the quantitative use of Western blotting, to determine its applicability and limits depending on the detection method and the data normalisation strategy used to quantitatively compare biological replicates of the same experimental conditions.Ī requirement for the quantitative use of Western blot data is the linearity between quantified intensities and protein amounts. Īlthough technical aspects of Western blotting have improved over the years, for example by extending the linear range of detection, it is not yet clear how much quantitative information can be obtained and in which settings. Two main applications are the parameterisation and validation of mathematical models of biological systems and the testing of statistical significance between two or more experimental conditions or treatments. Although originally a qualitative or at best a semi-quantitative method, with the rise of computational systems biology, Western blotting has become increasingly important for fully quantitative applications. Western blotting or protein immunoblotting, was introduced at the end of the 1970s to enable the detection of specific proteins. These results will aid users of Western blotting to choose a suitable normalisation strategy and also understand the implications of this normalisation for subsequent hypothesis testing. This causes the effect of normalisations by sum or optimal alignment on hypothesis testing to depend on the mean of the data tested for high intensity points, false positives are increased and false negatives are decreased, while for low intensity points, false positives are decreased and false negatives are increased. Normalisation by sum or by optimal alignment redistributes the raw data uncertainty in a mean-dependent manner, reducing the CV of high intensity points and increasing the CV of low intensity points. Analysis of published experimental data shows that choosing normalisation points with low quantified intensities results in a high normalised data CV and should thus be avoided. Thus, in the context of hypothesis testing, normalisation by fixed point reduces false positives and increases false negatives. Normalisation by fixed point tends to increase the mean CV of normalised data in a manner that naturally depends on the choice of the normalisation point. We consider how these different strategies affect the coefficient of variation (CV) and the results of hypothesis testing with the normalised data. Here we evaluate three commonly used normalisation strategies: (i) by fixed normalisation point or control (ii) by sum of all data points in a replicate and (iii) by optimal alignment of the replicates. To ensure accurate quantitation and comparability between experiments, Western blot replicates must be normalised, but it is unclear how the available methods affect statistical properties of the data. Western blot data are widely used in quantitative applications such as statistical testing and mathematical modelling.
